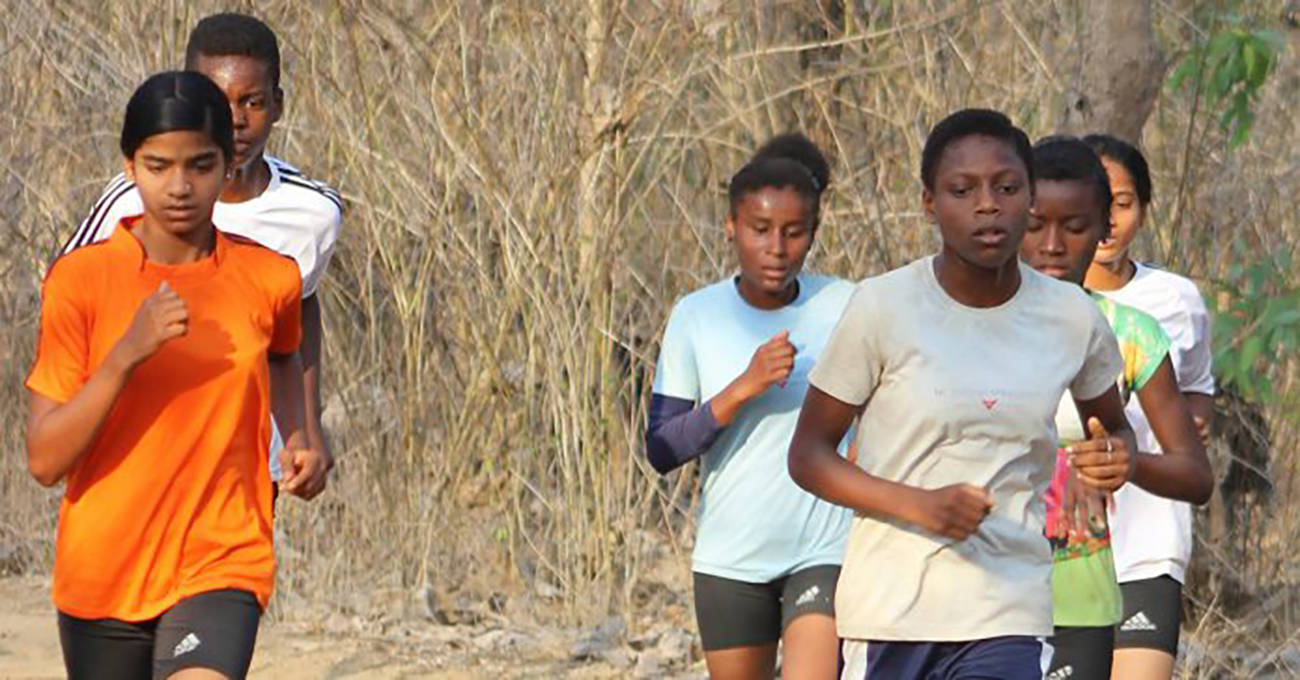
Winning with Data: Optimisation of training in sports
Indian Olympian swimmer Shikha Tandon is presently the director of US-based organisation Silicon Valley Exercise Analytics (SVEXA), which works on the role of data and technology driving an athlete’s performance. She was the first Indian to be employed in the prestigious US Anti Doping Agency. We present a new series of articles “Winning with data” by Shikha and her team in California. This is the first article of the series.
The 10,000 hours of practice rule, popularised by Malcolm Gladwell, is a commonly held belief to achieve expertise in any skill. While this claim has been debunked over the years, in the sports context, many athletes and coaches still believe that longer and harder training hours are the keys to success. However, the actual situation is much more complex. A crucial aspect is the individualisation of the training to match each athlete’s profile and potential, and to do so over time in all different situations. This article series describes scalable concepts for optimised training in sports. As the term suggests, the goal of optimisation is to make the efforts of the athlete as effective as possible, irrespective of the length and intensity of the training session.
In the previous Tokyo Olympics, held in 1964, the three men on the podium of the 5000m race finished the race within one second of each other. However, each of these men pursued vastly different training regimens in preparation for the race. The gold medalist focused on shorter harder interval sessions during his training, the silver medalist practiced high volume training with mainly long slow runs, and the bronze medallist was a proponent of a polarized training regime, i.e. mixing shorter harder interval sessions with long slow runs. As clearly shown by the results, all programs lead to world-class performance, but it is unknown if they would have been as good if they swapped training programs.
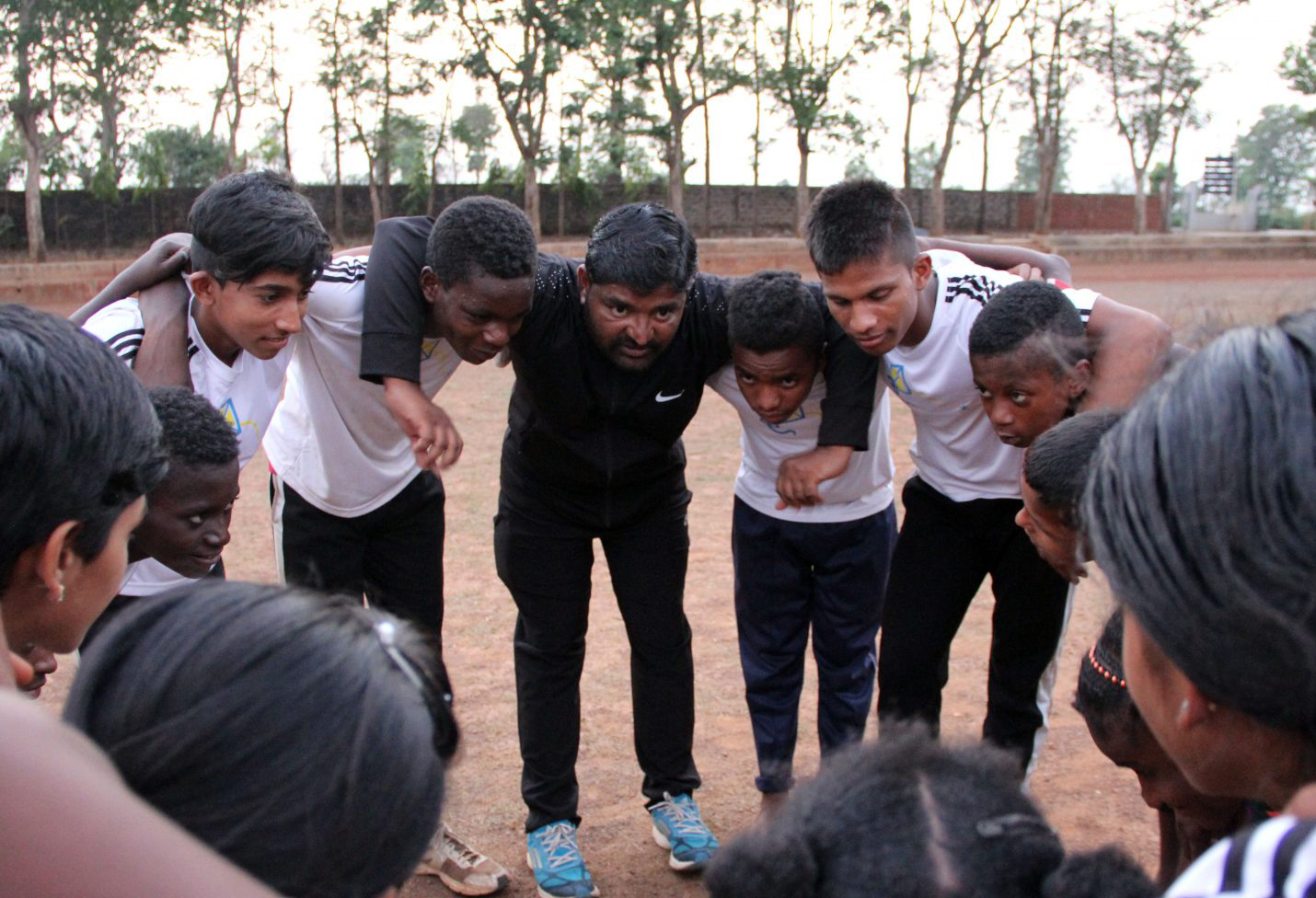
individualised training
Any coach, who has ever trained a group of people, elite or recreational, has probably observed that everyone doesn’t respond to the same training in exactly the same way. It is important to modify training regimens to suit the specific needs of each individual. Fortunately, we are now entering an era where truly individualised training optimisation is becoming possible. The sports industry has only very recently started to leverage the technological explosion and the leap forward in data science with developed deep learning and other artificial intelligence techniques.
While the fact of individual differences has been known for centuries, everyday practice in most sports and most clubs treat all athletes within a group in the same manner. In a scarily high number of cases, every player in a team is exposed to the same training session. For example, in a soccer team, some players might have to run twice as far in matches compared to others, while another group of players experiences much higher physiological loads in terms of high intensity running distance or number of accelerations and decelerations. A third group may have stayed on the bench in recent games, and a fourth group may have recently recovered from a minor illness or injury. However, each of these players, even those within the same group, experience the same training session with limited personalisation based on their specific circumstances. The reasons usually given are lack of time and resources and sometimes lack of knowledge.
An experienced coach, with a small group of elite athletes, is able to work closely with each member and have detailed daily conversations with them. The insights gained through this interaction allows the coach to perform a certain level of manual analysis and provide individualised training modifications for each athlete. However, that is not scalable for larger groups and teams, and most people definitely don’t have access to daily contact with top coaches. There is an inherent need to develop a system that automates a part of this process, which aids the coach to make more informed decisions for each athlete on the team. Such a system would help not only elite athletes pushing themselves to peak performance but also the recreational athlete training for a few hours a week. In an ideal world, these few hours of training would be optimised to focus on the things that give the best improvements for that specific individual.
So, how does optimisation work?
The first level of individualisation takes into account the specific demands of the sport and exposure to training and competition. The next level layers in the athlete’s individual profile. Each athlete responds in a uniquely different manner to the same training regimen. This personal response could also vary for the same individual on different days, depending on additional factors such as sleep and nutrition. An athlete that shows rapid and large improvement in response to a training program is traditionally interpreted by the coach as being gifted, whereas another athlete showing smaller improvements might be considered as untalented. However, this training response should be more accurately viewed as highly individual sensitivity to the given stimuli. If the athlete does not respond to the training, how often do we stop to question the stimuli, versus assuming it’s the athlete and their efforts?
If we know enough about the athlete’s profile, training load, and recovery status it’s possible to calculate the optimal training plan and training load for each day. Up until just a few years ago, the technology to in a practical way measure and monitor many of these variables did not exist. Everyone could whitewash the lack of individualisation by lacking the data and hard facts. Today, the data collection no longer poses a problem, with for example GPS watches, sleep tracking rings, and biomarkers in sweat or saliva. In most cases, the challenge is instead to translate the data tsunami into actionable insights.